HOSPITAL READMISSIONS SQL PROJECT
- Denzel Harry
- May 10, 2023
- 8 min read
This data analysis project takes a dive into hospital data by creating queries in PostgreSQL to extract insights in order to give suggestions on ways to optimize hospital performance and save money in the process.
This project uses an anonymized dataset sample from a hospital. This hospital has a strong incentive to reduce medical expenses for the members of its Medicaid Accountable Care Organization (MACO), the hospital receives a budget for its MACO members and must ensure their medical expenses do not exceed that amount.
Inpatient services account for a significant share of the MACO’s total medical expenses:
The hospital believes there is substantial opportunity to reduce unnecessary or preventable inpatient stays while still maintaining high-quality care.
My job as the Data Analyst was to solve the problem of figuring out what the cost drivers were for members of the Medicaid Accountable Care Organization and to calculate the readmission rate for these individuals as well. I was also tasked with completing a descriptive analysis on the members of the MACO as well as finding out what their inpatient stay information looks like.
Lets begin.
There were two tables we used. The first table 'maco' only had details of MACO members which included columns on the patient's memberid, sex, race, disability(if any), and age.
SELECT *
FROM maco

The second table 'ipclaims' had inpatient claim details for the MACO members and non MACO members. The columns of this table included memberid, discharge facility, discharge facility type, admission diagnosis group, admission date, discharge date, and paid amounts per visit.
SELECT *
FROM ipclaims
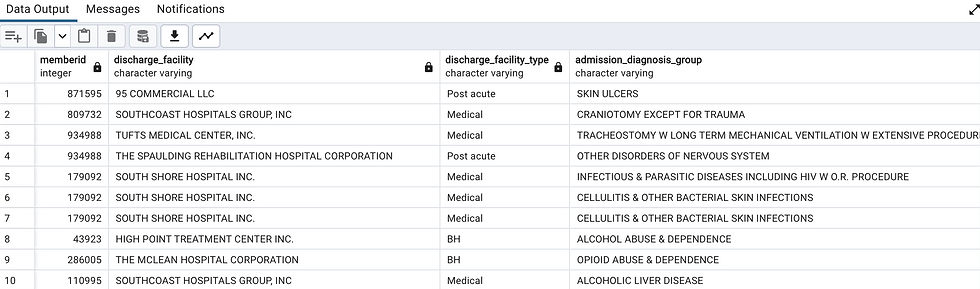
I started off doing a descriptive analysis for the inpatient stays filtering for the MACO members only.
SELECT admission_diagnosis_group, COUNT(admission_diagnosis_group)
FROM ipclaims
WHERE memberid IN (SELECT memberid
FROM maco)
GROUP BY admission_diagnosis_group
ORDER BY COUNT(admission_diagnosis_group) DESC
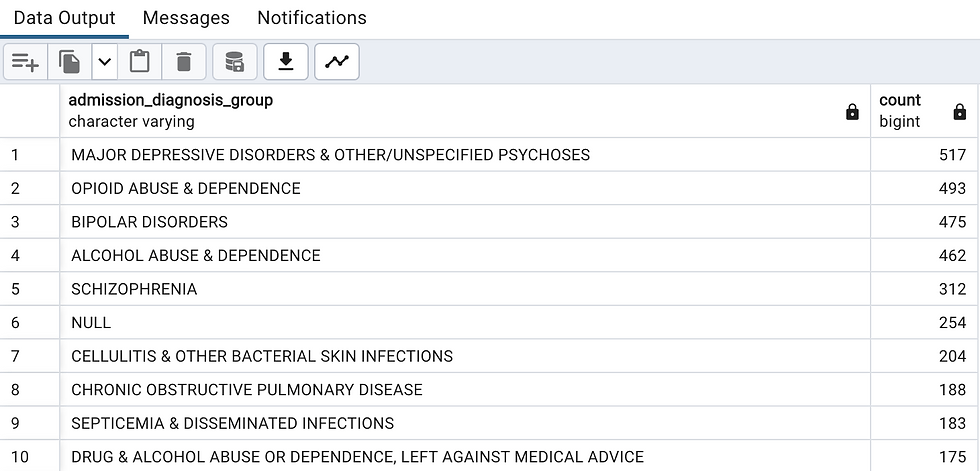
I created the query above to take a look into the iplclaims table to create a count and aggregate at the admission diagnosis groups. I used a subquery in the where statement to filter for only members of the MACO since the ipclaims table includes non MACO members. In the output above we see the top 5 most common admission diagnosis reasons for MACO impatient members have to do with drug/alcohol abuse and mental health disorders.
I wanted to take a quick glance at MACO members with higher than average paid amounts and just scan through quickly what the diagnosis' were.
SELECT maco.memberid, ipclaims.admission_diagnosis_group, ipclaims.paid_amount
FROM maco
LEFT JOIN ipclaims
ON maco.memberid = ipclaims.memberid
WHERE ipclaims.paid_amount >= (SELECT AVG(paid_amount)
FROM ipclaims
WHERE memberid IN (SELECT memberid
FROM maco))
ORDER BY paid_amount DESC
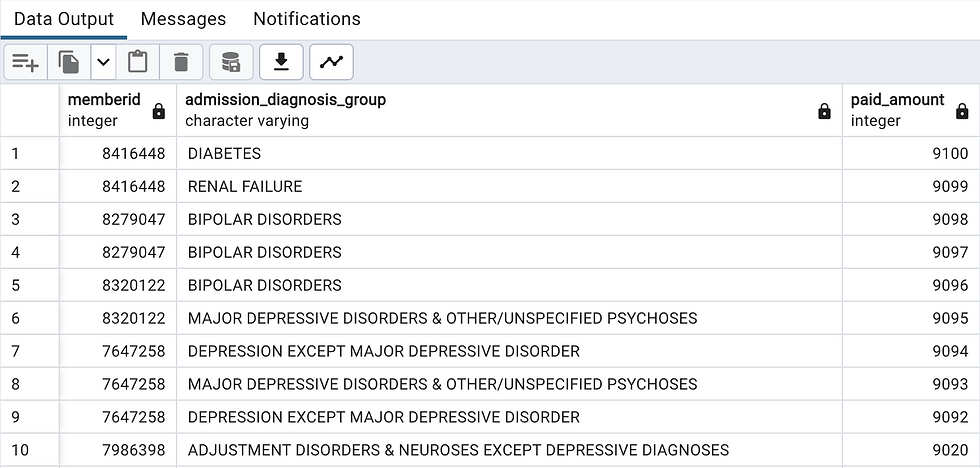
In the above query I left joined the ipclaims table on the maco table since I only wanted to look at the MACO members' claims. I also used a nested query within a nested query to correctly filter for the greater than average paid amounts of MACO members. A quick glance at the results of this query show trends similar to the query before this, showing higher paid amounts towards substance abuse and mental health disorders.
I wanted to take a quick glance and see where majority of these MACO inpatients typically are staying.
SELECT discharge_facility, COUNT(discharge_facility)
FROM maco
LEFT JOIN ipclaims
ON maco.memberid = ipclaims.memberid
GROUP BY discharge_facility
ORDER BY COUNT(discharge_facility) DESC

This marks the end of the descriptive analysis portion regarding inpatient stays for the ACO members.
Next, I wanted to do some descriptive analysis for the MACO members themselves.
I first started by looking at the percentage of BACO that was disabled.
SELECT
CAST(SUM(CASE WHEN disabled = 'Y' THEN 1 ELSE 0 END) AS FLOAT)/
CAST(SUM(CASE WHEN disabled = 'N' or 'Y' THEN 1 ELSE 0 END) AS FLOAT) AS disabledpopulation
FROM maco

I used a CASE Statement to find the percentage of the disabled population of the MACO members. We see that MACO only has about 10% of members that are disabled. I had to cast as FLOAT because the results were coming up as 0 without the cast.
I wanted to see who the people are that make up the MACO table.
SELECT sex, race, disabled, COUNT(*) AS countall
FROM maco
GROUP BY sex, race, disabled
ORDER BY COUNT(*) DESC
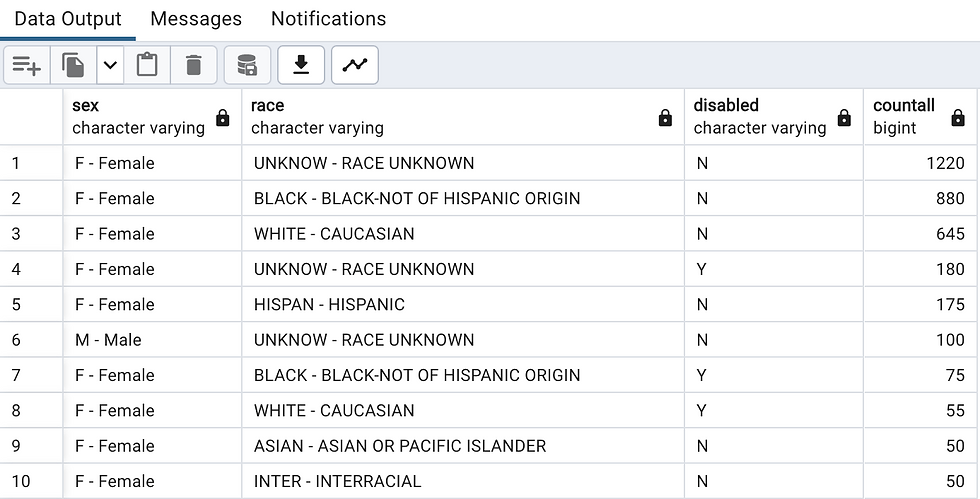
Aggregated by sex, race, and disability and we see that non disabled females of unknown race make up over 30% of MACO members. We also see that non disabled black females come in a close second.
I wanted to see the average age for the members that would be a part of each GROUP BY aggregate above but a GROUP BY wouldn't allow that so I had to create a partition.
I wanted to see the average age of all MACO members AND the average age for the specific aggregates we did above.
SELECT sex, race, disabled, avgagepart, averageageoverall, srdcounts
FROM
(SELECT sex, race, disabled,
ROUND(AVG(age) OVER(), 2) AS averageageoverall,
ROUND(AVG(age) OVER (PARTITION BY sex, race, disabled), 2) AS avgagepart,
ROW_NUMBER() OVER (PARTITION BY sex, race, disabled) AS srdfilt,
COUNT(*) OVER (PARTITION BY sex, race, disabled) AS srdcounts
FROM maco) AS subq
WHERE srdfilt = 1
ORDER BY srdcounts DESC

Though partitions are used to keep the identity of every single row, I still wanted to mirror the aggregate output that GROUPBY's do, only adding the average age(s) to the partition. I purposely created a partition that has an identical output as the previous query only with the exception of having average age per partition. I did this by creating a subquery and using the window function ROW_NUMBER to filter the rows in the outer query. This essentially would allow me to take away every row that belonged to the partition it was a part of, allowing me to get the specific output I wanted. Without this, I would still see the row results for the entire table, while this is typically what distinguishes a partition from a GROUPBY, I didn't wan't to use it that way for this specific query. We see that that average age is 27 years old and we see the results for averageage per partition.
This marks the end of the descriptive analysis on the MACO members.
Next, I wanted to find a solution to the cost drivers issue, which begins with finding out what the main cost drivers are for the inpatient stays of the MACO members.
SELECT admission_diagnosis_group, SUM(paid_amount) AS diagnosissums
FROM maco
LEFT JOIN ipclaims
ON maco.memberid = ipclaims.memberid
WHERE ipclaims.paid_amount >= (SELECT AVG(paid_amount)
FROM ipclaims
WHERE memberid IN
(SELECT memberid
FROM maco))
GROUP BY admission_diagnosis_group
ORDER BY SUM(paid_amount) DESC
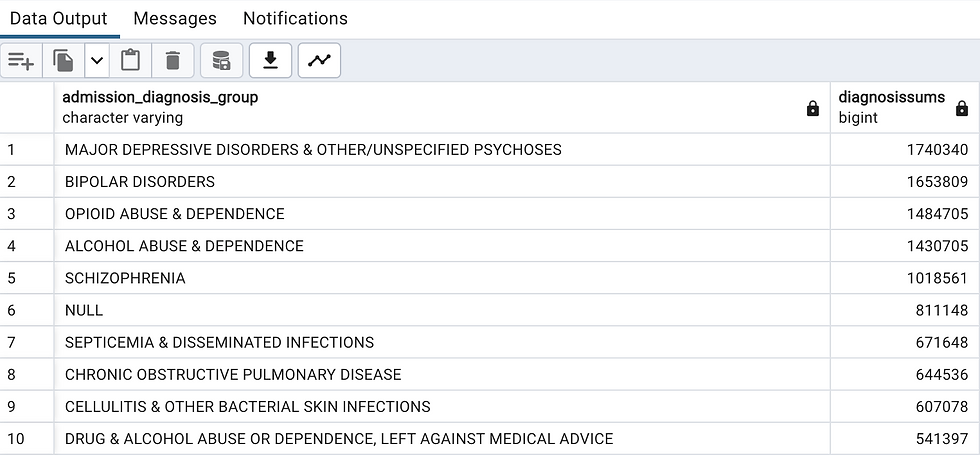
I summed the higher than average paid amounts of MACO members grouping it by admission diagnosis leading me to see that majority of the money being spent on MACO inpatient stays revolves around mental health, alcohol, and substance abuse problems.
The previous query filtered for higher than average paid amounts for inpatient stays for MACO members. I wanted to see the total sums to see if the results changed at all.
SELECT admission_diagnosis_group, SUM(paid_amount) AS diagnosissums
FROM maco
LEFT JOIN ipclaims
ON maco.memberid = ipclaims.memberid
GROUP BY admission_diagnosis_group
ORDER BY diagnosissums DESC
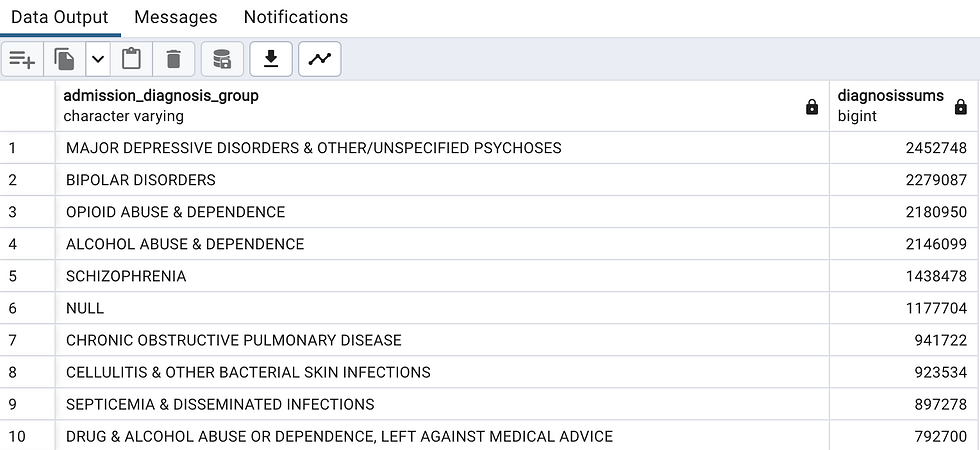
We see the full totals now and the top 5 admission diagnosis group's are still the same as the query before this.
My proposed business suggestion/solution for this is that if we could provide quality care to the patients dealing with mental health disorders/substance abuse problems without admitting them to stay in a bed, we could help the hospital save money.
Next we have the readmission rate calculation. This was especially difficult to complete, but I was able to successfully complete it.
I struggled with getting started so I looked to Stackoverflow for some assistance. I found a query to start with but had to modify it for my exact problem I was trying to solve and also the forum post was from a MySQL user and I'm on PostgreSQL so I had to do some transposing. This query below is what I started with.
WITH CTEROWS AS (
FROM
(SELECT bmcaco.memberid, admission_date, discharge_date,
ROW_NUMBER() OVER(PARTITION BY bmcaco.memberid ORDER BY bmcaco.memberid, admission_date) AS memadrow
FROM bmcaco
LEFT JOIN bmcipclaims
ON bmcaco.memberid = bmcipclaims.memberid) AS SUB
),
CTEDIFFS AS (
SELECT
FROM CTEROWS r1
LEFT OUTER JOIN cterows r2
ON r1.memandrow + 1 = r2.memandrow
WHERE r1.memberid = r2.memberid)
Essentially what I read from this base code is that we created a CTE and self joined it to itself in a way where the patient's next admission date could be referenced in the same row as the previous discharge date.
From there we selected the columns we wanted and created a case statement that compares the next admission date to the previous discharge date.
WITH CTEROWS AS (
SELECT maco.memberid, admission_date, discharge_date,
ROW_NUMBER() OVER(PARTITION BY maco.memberid ORDER BY maco.memberid, admission_date) AS memandrow
FROM maco
LEFT JOIN ipclaims
ON maco.memberid = ipclaims.memberid
),
CTEDIFFS AS (
SELECT r1.memandrow, r1.memberid, r1.admission_date, r1.discharge_date, r2.admission_date AS nextadmission,
date_part('day', r2.admission_date) - date_part('day', r1.discharge_date) AS dayssinceprevdischarge
FROM CTEROWS r1
LEFT OUTER JOIN cterows r2
ON r1.memandrow + 1 = r2.memandrow
WHERE r1.memberid = r2.memberid)
SELECT memberid, memandrow, admission_date, discharge_date, nextadmission, dayssinceprevdischarge,
CASE
WHEN dayssinceprevdischarge < 30 THEN 'READMITTED' ELSE 'No'
END AS Readmissionstatus
FROM CTEDIFFS
ORDER BY memberid, memandrow
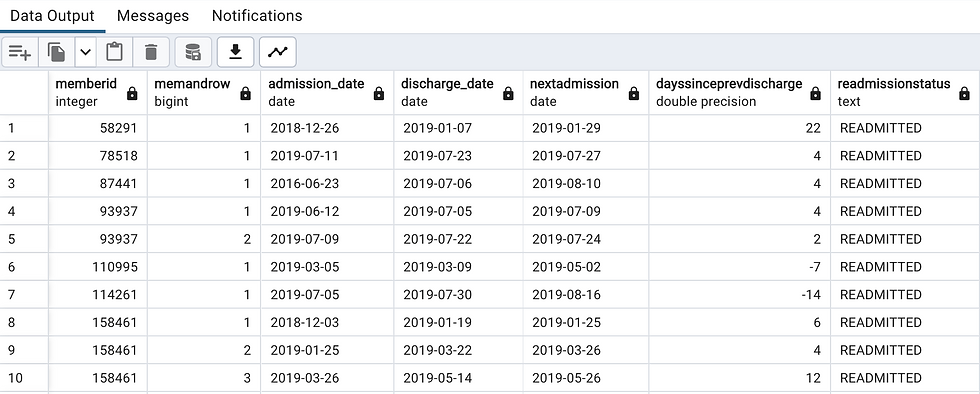
The way we partitioned the members and their dates, with this type of join, allowed us to offset the members' next admission to be on the same row as their previous discharge date. This then allowed us to create a case statement to compare the
next admission dates to the previous discharge date to see if it was in the 30 day range we were looking for.
The problem with the above query I found was the datepart formula didn't extract the day differences the way I wanted them to. Even some members that came back after 30 days would come up as -4 days even though it had been two months since they were last admitted. Some my nextadmission dates are outside of discharge time of 30 days and it still came up as negative, making the CASE statement incorrect. So I got rid of the datepart calculation and created my own case statement to correctly find the readmissions.
I created a case statement that took the nextadmission column value and compared it to the discharge date, setting a boundary of 30 days. If it was outside of the 30 day mark, the case statement would let me know.
WITH CTEROWS AS (
SELECT maco.memberid, admission_date, discharge_date,
ROW_NUMBER() OVER(PARTITION BY maco.memberid ORDER BY maco.memberid, admission_date) AS memandrow
FROM maco
LEFT JOIN ipclaims
ON maco.memberid = ipclaims.memberid
),
CTEDIFFS AS (
SELECT r1.memandrow, r1.memberid, r1.admission_date, r1.discharge_date, r2.admission_date AS nextadmission
FROM CTEROWS r1
LEFT OUTER JOIN cterows r2
ON r1.memandrow + 1 = r2.memandrow
WHERE r1.memberid = r2.memberid)
SELECT memberid, memandrow, admission_date, discharge_date, nextadmission,
CASE
WHEN nextadmission BETWEEN discharge_date AND (discharge_date + INTERVAL '30 DAYS') THEN 'readmitted'
ELSE 'NOTREADMITTEDWITHIN30DAYS'
END
FROM CTEDIFFS
ORDER BY memberid, memandrow

I was successfully able to find all of the patients that were readmitted.
I created a temp table for the readmissions since the CTE was getting messy.
CREATE TEMP TABLE readmissions
(memberid integer,
memandrow integer,
admission_date date,
discharge_date date,
nextadmission date,
readmissionstatus varchar)
INSERT INTO readmissions
WITH CTEROWS AS (
SELECT maco.memberid, admission_date, discharge_date,
ROW_NUMBER() OVER(PARTITION BY maco.memberid ORDER BY maco.memberid, admission_date) AS memandrow
FROM maco
LEFT JOIN ipclaims
ON maco.memberid = ipclaims.memberid
),
CTEDIFFS AS (
SELECT r1.memandrow, r1.memberid, r1.admission_date, r1.discharge_date, r2.admission_date AS nextadmission
FROM CTEROWS r1
LEFT OUTER JOIN cterows r2
ON r1.memandrow + 1 = r2.memandrow
WHERE r1.memberid = r2.memberid)
SELECT memberid, memandrow, admission_date, discharge_date, nextadmission,
CASE
WHEN nextadmission BETWEEN discharge_date AND (discharge_date + INTERVAL '30 DAYS') THEN 'readmitted'
ELSE 'NOTREADMITTEDWITHIN30DAYS'
END
FROM CTEDIFFS
ORDER BY memberid, memandrow
The temp table was successfully created.
SELECT *
FROM readmissions

I then created a CASE statement that calculates the counts to see how many readmissions there were.
SELECT
SUM(CASE WHEN readmissionstatus = 'readmitted' THEN 1 ELSE 0
END) AS readmittedcount,
SUM(CASE WHEN readmissionstatus = 'NOTREADMITTEDWITHIN30DAYS' THEN 1 ELSE 0 END) AS notreadmittedcount,
SUM(CASE WHEN readmissionstatus IN ('readmitted',
'NOTREADMITTEDWITHIN30DAYS') THEN 1 ELSE 0 END) AS totaladmits
FROM readmissions
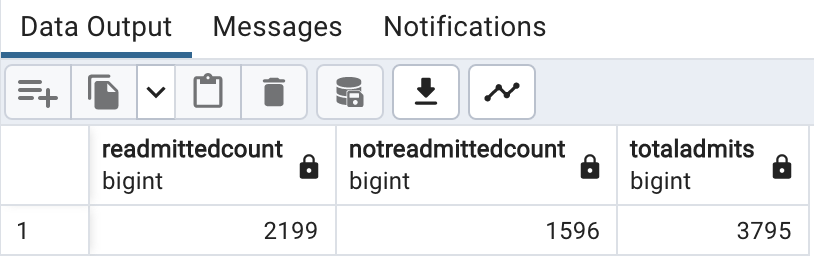
We see the readmission count is 2199. All that's left to do is find the denominator which would be a count of the discharge dates for the MACO members.
SELECT COUNT(discharge_date)
FROM ipclaims
WHERE memberid IN (SELECT memberid
FROM maco)
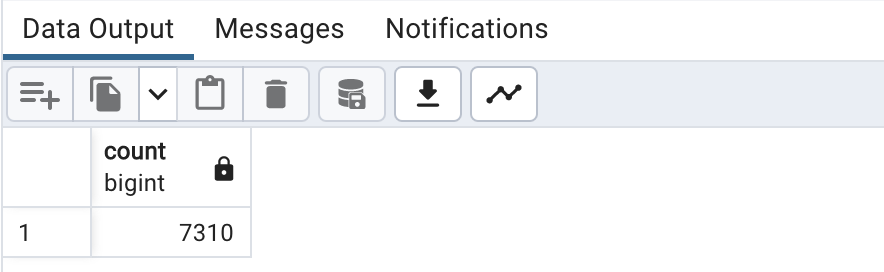
2199(readmission amount)/7310(total discharges) ~ 30% readmission rate
The average readmission rate for hospitals is around 15% so we see that this hospital has a higher than average readmission rate which is not a good thing.
Throughout this project we got the opportunity to take a look at the MACO members themselves and the main problems that the members are dealing with which cost the hospital a lot of money.
My proposed suggestion to the problem with MACO members is providing quality care to the patients dealing with mental health disorders/substance abuse problems without admitting them to stay in a bed. If we could complete that we could help the hospital save money.
I also believe that if we could take a closer look at the hospital discharge process for these members we could also lower the readmission rate, which is currently higher than average.
This completes my analysis for the hospital. Thank you for reading.
コメント